

Clarify will help data scientists detect statistical bias across the machine learning workflow and explain the models’ predictions. Data engineers can use this tool to detect bias across the models and build transparent, fair, and bias-free models. SageMaker Clarify addresses the on-going AI bias issues in machine learning models. Developers can use Pipelines to easily re-run an end-to-end workflow from SageMaker Studio using the same settings to get the same model or re-run the workflow with new data inputs for a new, updated model. SageMaker Pipelines is a workflow management and automation toolkit that will enable developers to log each step of an end-to-end machine learning workflow. Since the feature store resides in SageMaker Studio, it provides single-digit millisecond latency for inference. SageMaker Feature Store is a repository that enables developers to easily store, update, retrieve, and share machine learning features for training and inference.
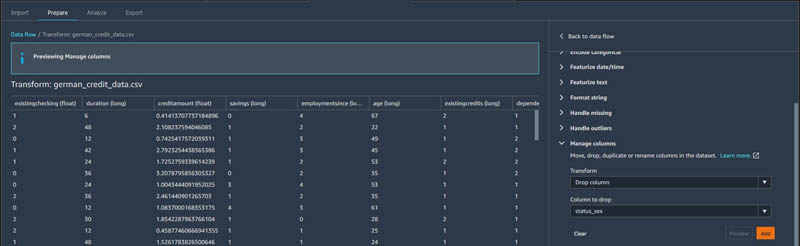
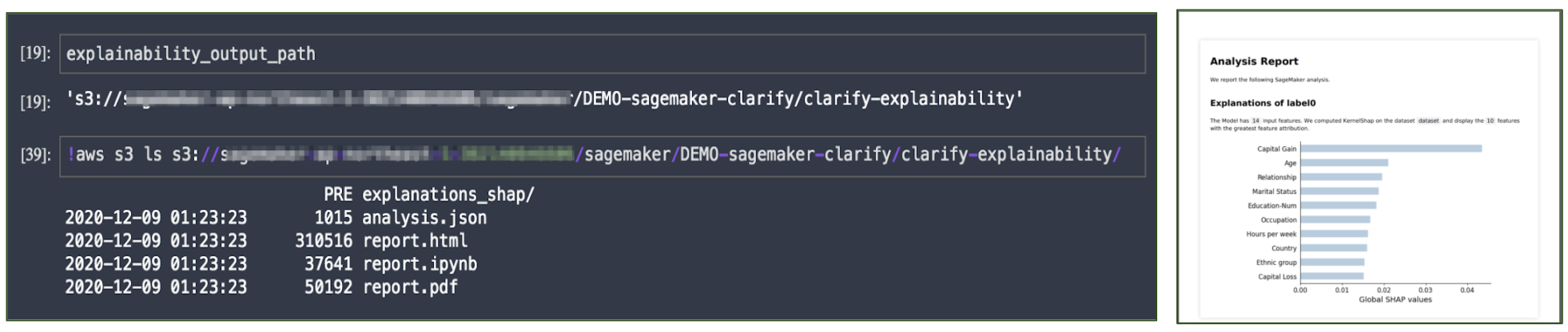
Customers can preview and inspect these transformations in SageMaker Studio and reuse the engineered features in SageMaker Feature Store. It contains over 300 built-in data transformers that can help customers normalize, transform, and combine features without writing any code. With this, developers can prepare data for training machine learning models faster. The company has added new features to the Amazon SageMaker platform, a machine learning service for developers to automate and scale their end-to-end machine learning workflow.ĭata Wrangler is a new service that automates data preparation and feature engineering (transforming data into features). Amazon Announced New SageMaker Capabilities Let’s find out this week’s major announcements. The company announced new SageMakers capabilities, a data lake for the healthcare industry, and a host of big data announcements. This week, the cloud behemoth focused on various machine learning and big data-related launches. Let’s look at the major announcements from the second week of the event.ĪWS re:Invent 2020, a cloud computing event hosted by AWS for the global cloud community, entered into its second week with several launches and informative sessions. Their definitions, see Amazon SageMaker Clarify Terms for Bias andįor additional information about post-training bias metrics, see Learn How Amazon SageMaker Clarify Helps Detect Bias and Fairness Measures for Machine Learning in Finance.The second week of AWS re:Invent 2020 saw a host of machine learning and big data products. Not be due to a machine learning model, but might still be detectable by post-trainingĪmazon SageMaker Clarify tries to ensure a consistent use of terminology. Group, referred to as a less favored facet d, experiences anĪdverse effect even when the approach taken appears to be fair. For example, the US concept of disparate impact that occurs when a There are legal concepts of fairness that might not be easy to capture because theyĪre hard to detect. Requiring different bias metrics to measure. There are different notions of fairness, each You assess performance by analyzing predicted labels or byĬomparing the predictions with the observed target values in the data with respect to These analyses take into consideration the data, including the labels, and Post-training bias analysis can help reveal biases that might have emanated fromīiases in the data, or from biases introduced by the classification and predictionĪlgorithms.
